AGI's impact on work.
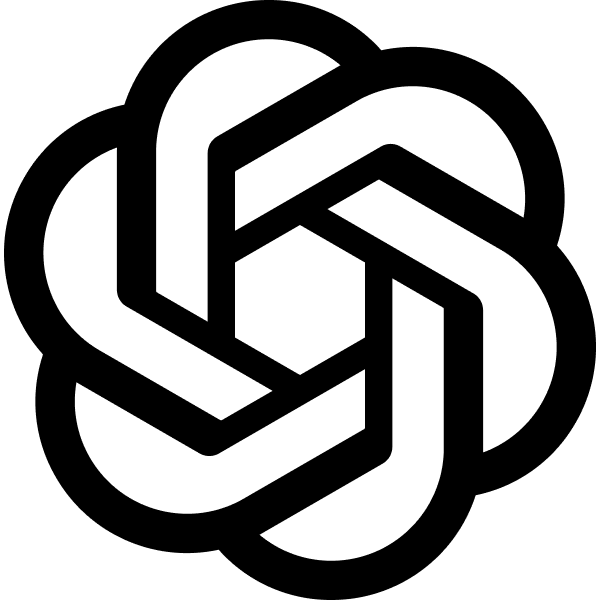
TODO: collapasable, copyable, download, etc
I don’t understand how AGI would make it so that no humans have to work. Artificial General Intelligence (AGI) refers to a type of AI that has the ability to understand, learn, and apply knowledge across a wide range of tasks at a human-like level. The idea behind AGI potentially making it so that humans don't have to work is based on the premise that it could perform most jobs as effectively, or even more effectively, than humans.
Here are several ways AGI could contribute to reducing or eliminating the need for human labor:
Automation: AGI could automate many jobs, particularly repetitive, manual, or labor-intensive tasks. This includes jobs in manufacturing, agriculture, transportation, and logistics. With advanced AGI, even complex cognitive tasks, such as writing, research, and decision-making, could be automated.
Problem-solving: AGI could solve complex problems that would typically require a team of experts, streamlining processes, and reducing the need for human intervention. This could include tasks in engineering, medicine, finance, and other areas that involve intricate problem-solving.
Creativity and innovation: AGI has the potential to be creative and innovate at a level equal to or beyond human capability. It could generate new ideas, designs, and solutions that would lead to more efficient systems and technologies, further reducing the need for human labor.
Personalized education and training: AGI could enable personalized education and training, allowing people to learn new skills quickly and efficiently. As a result, individuals might be able to transition between different types of work more easily, reducing the need for large numbers of people in any given occupation.
However, it is important to note that the idea of AGI making work obsolete for humans is speculative and relies on several assumptions. For instance, it assumes that AGI will reach a level of sophistication that allows it to perform all tasks, that society will embrace and adopt AGI technologies in all sectors, and that ethical, legal, and regulatory concerns will be addressed.
Even if AGI does result in widespread automation, there may still be roles for humans in society. People might focus on activities that emphasize uniquely human qualities, such as empathy, artistic expression, or providing care and companionship. In addition, new types of jobs may emerge that we cannot yet foresee.
Ultimately, the impact of AGI on work will depend on how it is developed, implemented, and integrated into society. It has the potential to significantly reduce the need for human labor, but it is up to policymakers, businesses, and individuals to shape the future of work in a way that benefits everyone.How would AGI automate fixing a plumbing issue in someone’s house?To understand how AGI could automate fixing a plumbing issue in someone's house, let's break down the process into several steps:
Problem identification: AGI would first need to identify the plumbing issue. Homeowners could report problems through an AI-enabled communication system, or AGI could analyze data from sensors installed in the plumbing system to detect issues proactively.
Diagnostics: Once the problem is identified, AGI would need to diagnose the issue. It could analyze data from the sensors, review images or video feeds, and compare the information to its vast knowledge of plumbing systems to determine the cause of the problem.
Solution development: With the diagnosis complete, AGI would determine the best course of action to resolve the issue. It would take into account factors such as the specific plumbing system, the severity of the problem, and the availability of resources to develop an appropriate solution.
Execution: To carry out the repair, AGI would either control a specialized robot or a team of robots designed for plumbing tasks. These robots would have the necessary tools and capabilities to access the plumbing system, remove damaged parts, and install replacements or make adjustments as needed. AGI would guide the robots throughout the process, ensuring that the repair is completed efficiently and effectively.
Quality assurance and follow-up: After the repair is completed, AGI would perform a series of checks to ensure that the issue has been resolved correctly. It could analyze sensor data, conduct pressure tests, and review images or video feeds to verify that the plumbing system is functioning as intended. If necessary, AGI could also schedule follow-up visits or maintenance to ensure the long-term reliability of the repair.
This scenario illustrates how AGI could potentially automate fixing a plumbing issue. However, it's important to note that we have not yet reached a level of AGI or robotics technology that can fully realize this vision. There are still many challenges to overcome, including refining AGI capabilities, developing advanced robotics systems, and addressing legal, ethical, and safety concerns.What are the biggest barriers in robotics technology that need to be solved before it can accomplish these tasks?Several significant challenges and barriers in robotics technology need to be addressed before robots can effectively perform complex tasks like plumbing repairs in a real-world setting. Some of these challenges include:
Dexterity and manipulation: One of the biggest challenges is enabling robots to handle objects and tools with the same level of dexterity and precision as a human. Robots need to be able to grasp, manipulate, and position objects accurately and delicately, as well as adapt to variations in size, shape, and texture.
Perception and understanding: Robots need to be able to perceive their environment accurately and make sense of it to execute tasks effectively. This includes understanding and interpreting visual, auditory, and tactile data, as well as recognizing and dealing with unexpected situations or obstacles.
Navigation and mobility: For robots to operate in complex environments like a home, they need to navigate and move around safely and efficiently. This requires the ability to plan and execute paths through cluttered spaces, avoid obstacles, and adapt to changing conditions such as uneven surfaces or dynamic objects.
Adaptability and learning: Real-world environments are highly variable, and robots need to be able to adapt to new situations and learn from experience. They must be able to adjust their behavior based on context, learn from their mistakes, and incorporate new information to improve their performance over time.
Human-robot interaction: For robots to work effectively in human environments, they need to be able to understand and respond to human cues, such as speech, gestures, and facial expressions. They should also be able to communicate their intentions clearly and collaborate with humans effectively.
Energy efficiency and autonomy: Robots need to be energy-efficient and have a sufficient operational time to be practical for various tasks. They should also be able to operate autonomously, without constant human supervision, and make decisions based on their understanding of the environment and task requirements.
Safety and reliability: Ensuring the safety and reliability of robots is crucial, especially when they are working in close proximity to humans or dealing with critical infrastructure. Robots need to be designed with safety features and fail-safe mechanisms to prevent accidents and minimize risks.
Cost and scalability: For widespread adoption, robots need to be affordable and easily scalable. This includes reducing the cost of hardware and maintenance, simplifying the deployment process, and developing standardized components and software platforms that facilitate interoperability.
Addressing these challenges will require advancements in various areas, including artificial intelligence, materials science, computer vision, control systems, and human-robot interaction. Collaborative efforts among researchers, engineers, and policymakers will be crucial in overcoming these barriers and enabling the development of more capable and versatile robotic systems.How could AGI solve the energy crisis?While AGI itself may not directly solve the energy crisis, it could contribute significantly to addressing the issue by improving energy efficiency, optimizing resource allocation, and accelerating the development of new technologies. Some of the ways AGI could help solve the energy crisis include:
Optimizing energy production: AGI could optimize the operation of power plants, including renewable energy sources such as solar and wind, by predicting fluctuations in energy demand, adjusting energy production accordingly, and managing energy storage systems.
Smart grid management: AGI could play a central role in managing smart grids, which are electricity networks that use digital technology to monitor and control the flow of energy. It could improve grid efficiency, balance supply and demand, and enhance the integration of renewable energy sources.
Energy-efficient design: AGI could help design more energy-efficient buildings, vehicles, and industrial processes by simulating and analyzing various design alternatives, considering factors like materials, insulation, and aerodynamics, and identifying the most efficient options.
Predictive maintenance: By analyzing data from sensors in power plants, electrical grids, and other energy infrastructure, AGI could predict equipment failures and maintenance needs, minimizing downtime and optimizing the performance of these systems.
Accelerating research and development: AGI could assist in researching and developing new energy technologies, such as advanced batteries, fusion power, and more efficient solar panels. It could analyze vast amounts of scientific data, identify promising research directions, and even generate new ideas and hypotheses.
Climate and environmental modeling: AGI could improve our understanding of the Earth's climate system and the impacts of various energy policies by creating more accurate and sophisticated climate models. This would enable better decision-making and help identify the most effective strategies for reducing greenhouse gas emissions and mitigating climate change.
Resource discovery and extraction: AGI could help identify new sources of energy, such as untapped oil, gas, or geothermal reserves, by analyzing geological data and identifying patterns that indicate the presence of these resources. It could also optimize extraction processes, making them more efficient and sustainable.
Personalized energy consumption: AGI could help individuals and organizations optimize their energy consumption by providing personalized recommendations based on their usage patterns, preferences, and available resources. This could include suggestions for energy-saving appliances, home automation systems, or changes in behavior.
It's important to note that the successful deployment of AGI in addressing the energy crisis would depend on the collaboration of governments, businesses, and individuals, as well as addressing ethical, legal, and regulatory concerns. By combining AGI's potential with well-coordinated efforts across different sectors, we can work toward more sustainable energy solutions and mitigate the impacts of the energy crisis.How could AGI solve the food production crisis?AGI could help address the food production crisis by increasing agricultural efficiency, reducing waste, optimizing resource allocation, and facilitating the development of new agricultural technologies. Here are some ways AGI could contribute to solving the food production crisis:
Precision agriculture: AGI could optimize farming practices by analyzing data from sensors, drones, and satellite imagery to provide real-time information on soil conditions, moisture levels, nutrient availability, and pest presence. This would enable farmers to make more informed decisions about irrigation, fertilization, and pest control, ultimately increasing crop yields and reducing resource use.
Crop and livestock management: AGI could help farmers select the best crops and livestock breeds for their specific conditions, taking into account factors like climate, soil type, and market demand. It could also optimize planting and harvesting schedules, as well as feeding and breeding practices, to maximize productivity and minimize waste.
Supply chain optimization: AGI could improve the efficiency of the food supply chain by predicting fluctuations in demand, optimizing transportation and storage, and reducing food waste. This would ensure that more food reaches consumers in a timely and cost-effective manner.
Climate-resilient agriculture: AGI could help develop new crop varieties and farming practices that are more resistant to climate change, pests, and diseases. By analyzing vast amounts of data on plant genetics, environmental conditions, and agricultural practices, AGI could identify the most promising strategies for increasing the resilience of our food production systems.
Alternative food sources: AGI could accelerate the development of alternative food sources, such as lab-grown meat or plant-based protein alternatives, by optimizing production processes and identifying the most efficient and sustainable methods for producing these foods.
Aquaculture and fisheries management: AGI could help optimize the management of aquaculture and wild fisheries by monitoring fish stocks, predicting changes in population dynamics, and recommending sustainable fishing practices. This would help ensure the long-term availability of fish as a food source while minimizing environmental impacts.
Policy and resource allocation: AGI could assist policymakers in making better decisions about agricultural subsidies, land use, and water management by analyzing the potential impacts of different policy options on food production, economic development, and environmental sustainability.
Education and training: AGI could provide personalized education and training to farmers, enabling them to adopt new technologies and practices more quickly and efficiently. This would help increase the overall productivity of the agricultural sector and ensure that farmers have the skills and knowledge needed to adapt to changing conditions.
While AGI has the potential to make significant contributions to solving the food production crisis, its successful implementation will depend on collaboration among governments, businesses, researchers, and farmers. Addressing ethical, legal, and regulatory concerns will also be crucial to ensure that AGI is used in a way that benefits everyone and minimizes potential negative consequences.What is the best ML model or technique for predictions from a dataset of 10,000 data points?There is no one-size-fits-all answer to which machine learning model or technique is the best for making predictions from a dataset of 10,000 data points. The optimal choice depends on various factors, such as the nature of the data, the type of problem you're trying to solve (classification, regression, etc.), and the specific requirements of your application (accuracy, interpretability, etc.).
Here are some general guidelines to help you choose an appropriate machine learning model or technique:
Start with exploratory data analysis: Before selecting a model, it's important to analyze your dataset and understand its characteristics, such as its distribution, correlation between features, and presence of outliers. This will help you determine which models might be suitable for your data.
Consider the problem type: Different models are suited to different types of problems. For example, if you're trying to predict a continuous target variable, you might consider regression models like linear regression, ridge regression, or support vector regression. For classification tasks, you could consider logistic regression, support vector machines, or decision trees, among others.
Assess model complexity: Balance the trade-off between model complexity and the risk of overfitting or underfitting. Simple models like linear regression or decision trees may work well for small datasets or when the relationships between features and the target variable are relatively straightforward. More complex models, such as deep learning or ensemble techniques like random forests and gradient boosting machines, may be more suitable for larger datasets or when the relationships are more complex.
Evaluate model performance: Use techniques like cross-validation to assess the performance of different models on your dataset. Compare performance metrics, such as mean squared error for regression tasks or accuracy, precision, and recall for classification tasks, to determine which model performs best on your data.
Consider interpretability: In some applications, it's important to understand the reasoning behind the model's predictions. If interpretability is a priority, you may want to choose a model that is more transparent, such as linear regression or decision trees, over more complex models like neural networks, which can be harder to interpret.
Regularization and hyperparameter tuning: Regularization techniques like Lasso and Ridge regression can help prevent overfitting by penalizing large model coefficients. Also, experiment with different hyperparameters to find the optimal settings for your chosen model.
Remember that no model is universally the best for all situations. You may need to try several models, evaluate their performance, and iterate on your approach to find the model that works best for your specific dataset and problem.
AGI's impact on work. created at Tue Jan 20 1970 10:24:21 GMT+0000 (Coordinated Universal Time)Related Chats
AGI's Impact on Capitalism 0.747
Human Light Cone AGI. 0.478
AI Workshop: Missing, Improved, Removed 0.440
AI UI Designer Opportunity 0.381
Umbrella Issue Management 0.378
AI-Enabled Computers Incoming 0.376
Phone vs. Special Devices 0.369